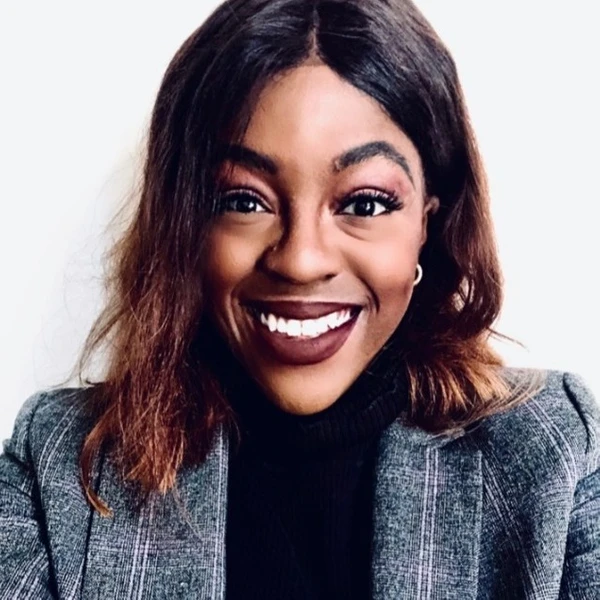
Reading
Business
I read books multiple times per week
Tiffany Tuedor
695
Bold Points1x
Nominee1x
Finalist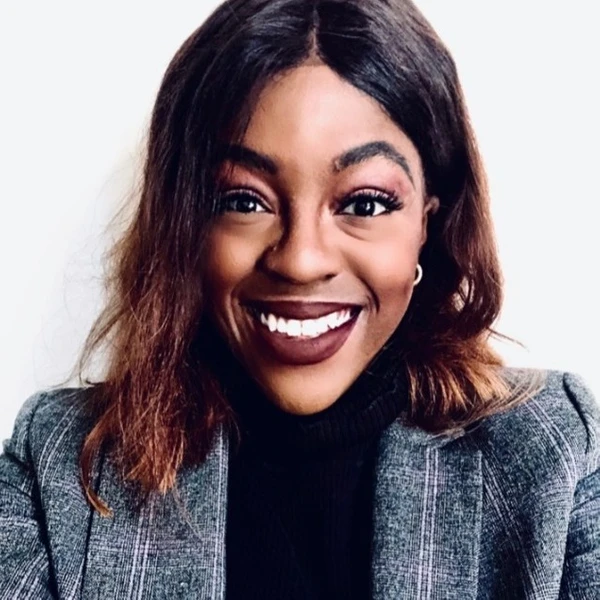
Tiffany Tuedor
695
Bold Points1x
Nominee1x
FinalistBio
Tiffany is an MSc Candidate at Harvard Medical School, studying Biomedical Informatics. She is passionate about evidence-based patient outcomes, using real-world data (RWD) to optimize clinical decision making and advance precision medicine. Tiffany uses her skills in data science & analytics, machine learning, biomedical & market research, and business development to address the latest challenges in medical diagnostics, through digital solutions.
Education
Harvard College
Master's degree programMajors:
- Bioinformatics
Columbia University in the City of New York
Bachelor's degree programMajors:
- Biomedical/Medical Engineering
Miscellaneous
Desired degree level:
Graduate schools of interest:
Transfer schools of interest:
Majors of interest:
Career
Dream career field:
Medicine
Dream career goals:
Data Scientist
Data Science Consultant
Accenture2017 – 20203 years
Sports
Rowing
Varsity2009 – 20134 years
Research
Bioengineering and Biomedical Engineering
Columbia University — Research Assistant2013 – 2015
Arts
- IllustrationPresent
Future Interests
Advocacy
Politics
Volunteering
Philanthropy
Entrepreneurship
Black Students in Tech Grant
I think my iPhone is cheating on me. Think about it. From my heart rate levels from 6AM workouts, Instagram knows to send me ads about ClassPass™; from my Apple Pay transactions, Amazon knows to recommend me trending skincare products; from the sentiment in my late-night texts to my best friend, Apple may not know who to give that information, but I’m telling you that I’m both anxious and excited about just starting grad school. Contrarily, it should behoove me to consider how these seemingly irrelevant fragments of information could inform a physician on my health. I would argue that these “fragments”, adding to the incessantly evolving vocabulary of “real-world data (RWD)”, are prompting physicians to blend our digital DNA signatures with our existing DNA, in tandem with other biomedical data, to detect changes in a patient’s health and optimize clinical decision making.
Therefore, I am excited to be in the Biomedical Informatics (DBMI) program at Harvard Medical School (HMS), to solve key challenges in clinical prognosis and diagnostics, powered by RWD, while advancing precision and translational medicine. By collaborating with organizations like the National Institute of Health’s (NIH) All of Us Research Program, I would particularly like to focus on digital phenotyping – what biomarkers (i.e. screen use, GPS, voice, etc.) can detect condition onset earlier? Through my time in the Harvard Biomedical Informatics Program, I will evaluate how clinical workflows and data ecosystems function, to discern how to infuse these non-traditional RWD sources mentioned into clinical settings, further expanding the digital footprint of medicine.
As for my background, I received my Bachelor’s degree in 2017, from Columbia University in the City of New York, where I studied Biomedical Engineering. Through my coursework and research at the Ultrasound Elasticity Imaging Lab (UEIL) in the Columbia University Medical Center (CUMC), I scrutinized how to best implement and innovate diagnostics methods in clinical settings. For example, for my Capstone project, our team’s goal was to craft a rapid, accurate, non-invasive solution to improve bacterial ear infection diagnostics for pediatric patients. When our initial hypothesis, which required acoustic reflectometry, a standard technique for detecting middle ear effusion, failed, I turned to machine learning as an alternate solution. I taught myself Python, given my familiarity with MATLAB, to develop a Support Vector Machine (SVM) Image Classification model, and Amazon Web Services (AWS), to 1) train my model with over 1000 images from a medical image library and 2) deploy my model from our Raspberry Pi 3 device that captured images of the patient’s eardrum. It was then that I began to explore the value of Machine Learning (ML) in clinical diagnostics.
Fast forward two years later, I’ve been crafting my skills in data science and ML to learn how to optimize clinical decision making and drive evidence-based outcomes. Through my certifications in Data Science and Machine Learning Engineering from General Assembly and Udacity, respectively, I have explored new data-driven solutions, such as detecting prescription opioid abuse from tweets, using the Twitter API, Natural Language Processing (NLP), and a Random Forest Classifier (RCF), and predicting hospital readmission for diabetic patients using Center of Medicare & Medicaid Services (CMS) data and decision trees. Enrolling in the Harvard DBMI program will grant me the opportunity to further understand biomedical data and deepen my data science skills, particularly in deep learning and NLP, in clinical settings.
I've worked as a Data Science Consultant in the Applied Intelligence (AI) practice, at Accenture Digital, applying my latest analytics skills to drive business decisions for Life Sciences clients in an industry setting. I was a Data Scientist and Life Sciences Lead in the Applied Intelligence (AI) Studio, in our NYC Innovation Hub. The purpose of our team is to apply analytics, using key tools like Python, R, Tableau, and SQL, to inspire our client’s growth strategies. For example, through tracking an individual’s over the counter (OTC) transactions at a pharmacy, their online browsing data, and prescription behavior, I could identify diabetic and pre-diabetic patient populations, illustrate patient experiences in a target geolocation, and recommend diabetic services that a top US-wide pharmacy should develop in 2020. Through the work in my program, I will be able to blend these 3rd party data sources with key clinical data points that would capture an even more holistic, 360-degree view of a patient’s lifestyle and needs, beyond the four walls of a doctor’s office.
So, in conclusion, to answer this question: What if they knew me like my iPhone did? My doctors, that is. I may have some hypotheses, but to be honest, I don’t have a specific answer yet. However, as a student in the Harvard DBMI program, through my passion for technology, medicine, behavioral economics, and business, I will: 1) leverage my background in biomedical engineering to identify pressing challenges impacting human health in our digital age; 2) apply my data science skills to craft data-driven solutions for these challenges, 3) apply my ML engineering skills to assess how to ingest RWD into my ML models, considering the fragmented EHR ecosystem, and 4) apply my skills in consulting and business development to translate my research, in the Clinical Decision Making and/or Artificial Intelligence research areas, into digital, innovative products in the Digital Health & MedTech industries. Through all these endeavors, not only could I potentially answer what if, but maybe when….
#BlackLivesMatter Scholarship
What if they knew me like my iPhone did? Think about it. From my heart rate levels and ClassPass™ activity, I work out almost every morning at 6:00AM; from my Apple Pay transactions, I spend an exorbitant amount on trending skincare products on Amazon; from the sentiment in my late-night texts to my best friend, I’m both anxious and excited about starting grad school this fall . It should then behoove me to consider how these seemingly irrelevant fragments of information could inform a physician on my health. I would argue that these “fragments”, adding to the incessantly evolving vocabulary of “real-world data (RWD)”, are prompting physicians to blend our digital DNA signatures with our existing DNA, in tandem with other biomedical data, to detect changes in a patient’s health and optimize clinical decision making.
So, let’s unpack an example on why we need to interweave various forms of RWD into clinical decision making: are there signs of melanoma that could be detected from an iPhone image, geolocation data – to track UV ray exposure & temperatures, and a classification model, before the human eye could? Why does this matter? Personally speaking, although grateful for all the melanin I inherited from my Nigerian roots, my hyperpigmentation is not entirely prioritized in medicine and research overall. Even though melanoma is 20X more common in Caucasians than in African-Americans, the estimated five-year melanoma survival rate for African-American patients is only 65%, versus 91% for Caucasians, proving that there may be some discrepancies in how patients are diagnosed, or treated, across ethnicities.
Especially as a black woman, I am concerned that missing data on racial demographics in public health databases and electronic health records (EHRs), perhaps due to lack of access to care, can inherently bias the ways that conditions are diagnosed in minorities. Even beyond melanoma, I am curious to investigate new machine learning (ML) methods and assumptions that could help impute missing data and better evaluate racial disparities in clinical decision making processes, through the power of RWD.
Therefore, I am excited about enrolling in the Biomedical Informatics (DBMI) program at Harvard Medical School (HMS), to solve key challenges in clinical prognosis and diagnostics, powered by RWD, while advancing precision and translational medicine. Through my time in the Harvard Biomedical Informatics Program, I will evaluate how clinical workflows and data ecosystems function, to discern how to infuse these non-traditional RWD sources mentioned into clinical settings, further expanding the digital footprint of medicine.
As for my background, I received my Bachelor’s degree in 2017, from Columbia University in the City of New York, where I studied Biomedical Engineering. Through my coursework and research, I scrutinized how to best implement and innovate diagnostics methods in clinical settings.
Fast forward two years later, I’ve been crafting my skills in data science and ML to learn how to optimize clinical decision making and drive evidence-based outcomes. I've worked as a Data Science Consultant in the Applied Intelligence (AI) practice, at Accenture Digital, applying my latest analytics skills to drive business decisions for Life Sciences clients in an industry setting. I believe that my education will enable me to understand key data sources and ecosystems that can capture an even more holistic, 360-degree view of a patient’s lifestyle and needs, beyond the four walls of a doctor’s office.
So, in conclusion, to finally answer this question: What if they knew me like my iPhone did? My doctors, that is. I may have some hypotheses, but to be honest, I don’t have a specific answer yet. However, through the Harvard DBMI program, through my passion for technology, medicine, behavioral economics, and business, I would like to: 1) leverage my background in biomedical engineering to identify pressing challenges impacting human health in our digital age; 2) apply my data science skills to craft data-driven solutions for these challenges, 3) apply my ML engineering skills to assess how to ingest RWD into my ML models, considering the fragmented EHR ecosystem, and 4) apply my skills in consulting and business development to translate my research, in the Clinical Decision Making and/or Artificial Intelligence research areas, into digital, innovative products in the Digital Health & MedTech industries. Through all these endeavors, not only could I potentially answer what if, but maybe when….